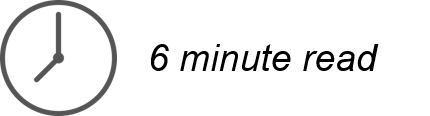
This is the first post in a series of three (probably) looking at a legal information project we’ve been working on. The project is to extract deal data points from SPAs (things like warranty periods and de minimis thresholds), analyse the data over a period and then present the results to end users in a digestible way.
This post will talk a little about collecting the data. The following posts will talk about:
- analysing ‘small’ datasets (which, I think, are far more common than ‘big’ datasets in law); and
- methods for delivering information to end users.
But first, let’s recap why we’re doing this. Fee-earners are often asked to give a steer as to “what’s market” for M&A deal terms. Maybe:
What’s the usual warranty period given by trade sellers in the tech industry?
This might be an explicit question that the client asks, or it might be implicit in suggesting a starting point for negotiation. The aim of the project is to help share the firm’s collective knowledge so that if fee-earners are coming to a new area, or want to understand if terms are changing over time, they can make use of our past experience.
With that in mind I wanted to suggest some points to think about when collecting data for a project like this. I’ll illustrate with SPA examples, but hopefully it will be generally relevant.
Aggregation means summarisation
One of the luxuries about working at Slaughter and May is there is lots of bespoke work. But non-standardised agreements mean fluffy concepts: we can draw up a list of the points we want to extract from an SPA but when you look at the documents you quickly find examples that don’t neatly fit into your list.
For example, you might start by thinking you just want to extract the warranty period. You will immediately run into examples where there are different periods for different types of warranties. And soon after that you’ll run into examples where there are different periods for different classes of seller.
You have a choice each time you meet a new type of example: either you expand your list (add a new column to your spreadsheet), or put it into an existing bucket, accepting that you are losing some detail. As lawyers, I think our natural instinct is to do the former – points of detail can be important and it’s our job to be across them. But if you don’t do any summarising, you won’t be able to give the high-level view; you won’t be able to write the executive summary. If you can’t resist recording the detail, make sure you are also categorising at a higher-level as you go.
Clean as you go
From a data perspective, there’s a world of difference between:
- Warranty period: “1 year”
- Warranty period: “First anniversary of the completion date”
- Warranty period: “15 March 2021”
The second one is annoying because someone has to manually change this to “1 year” before you can plot a graph, but the third one is potentially useless: if you don’t also capture the completion date, it is meaningless.
If you spend your life in Excel, this point will seem so obvious as to barely be worth making. But even if it is obvious to you, it may well not be obvious to the people collecting the data (many of whom chose a legal career in preference to something more quantitative).
So, be hyper clear about the form you want the information in and save yourself a job by having the reviewers report normalised data, as well as the raw input.
Future proof
In the future, people will ask new and different questions of your documents. No matter how comprehensive your data extraction process, people will ask questions that your data can’t answer. This is good and normal. There are a couple of things you can do to make your future life easier:
- Ensure that the data you have extracted can be tied back to the source documents.
That will ensure your data can be supplemented with new information, rather than creating disparate unconnected datasets. Ideally you’d achieve this by recording the data as part of the document’s wider record in your knowledge management system. If that’s not feasible, try at least to include a unique identifier for the document. Just including the file name of the document stored on your desktop doesn’t count.
- Record the purpose of your review with the data itself.
Include the scope, assumptions and limitations that applied to your project. That will help you determine whether it is actually appropriate to re-use the data for the new project, or if you should start from a new basis. For example, a project to establish market deal terms has a different focus (and makes different processing decisions) to a project to measure matter complexity.
If you would like to share your own experiences, learn more or get involved, please get in touch at LegalDesign@SlaughterandMay.com.
